The Codemakers
Herbie Bradley on building Britain’s AI advantage and what the AI Safety Institute can teach us about state capacity
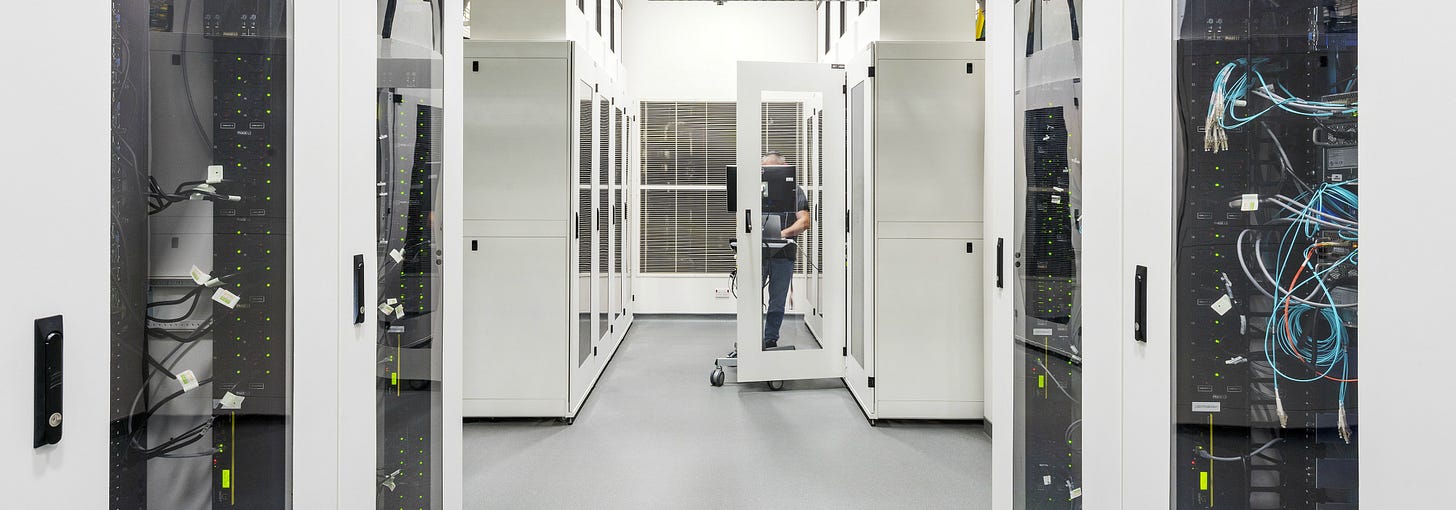
While the deficiencies in British state capacity are well documented, the AI Safety Institute (AISI) is an exceptional demonstration of how government can effectively build and scale something new. Among the international network of AISIs, Britain’s is the most well-resourced. Its employees earn prestigious nominations, win awards for their research, and present their work at leading conferences in the field. Their expertise has also become a valuable resource for the government on AI policy matters beyond safety, creating positive spillover effects for the rest of the ecosystem.
With the AI Opportunities Action Plan now moving from ink on paper to implementation, it is a good moment to remind ourselves that government can, in fact, do new things well.
Herbie Bradley, AI governance and policy expert and former member of the technical staff at the AISI, saw its growth from the Frontier AI Taskforce in the Department for Science, Innovation and Technology. During his time in government, Herbie was also involved in AI Research Resource (AIRR), national supercomputing infrastructure for AI research. We discussed how to ensure the government succeeds in AI, and the rewards on offer from doing so.
What we discussed
How could the upcoming Industrial Strategy help the UK to get ahead of the curve and leverage areas of its competitive advantage in AI?
What can the AISI teach us about building and scaling state capacity?
Is the AISI more of an advantage for the government, the British AI ecosystem, or both?
Do AI agents change anything about how countries should approach regulation?
What could an AI safety market look like, and where do untapped opportunities lie?
AISI theories of change and future missions
The risks and opportunities of putting the AISI on a statutory footing
Implications of the new Trump Administration for the UK AISI
Why does the UK need the AIRR, and how to realise its full potential?
Is the twenty-fold compute capacity increase that was recommended in the AI Opportunities Action Plan enough?
Lessons in building state capacity or launching a new, impactful initiative
Start a new team. Existing teams may become too entrenched in bureaucracy to move fast or be innovative enough.
Secure significant political backing. It helps with navigating bureaucratic hurdles, such as unconventional hiring processes.
Create performance incentives. These can be intrinsic motivation from team members believing in the initiative’s ideals, external pressures like tight deadlines, or competitive dynamics with other teams.
Hire externally. Recruit people from industry and the startup world who are accustomed to moving quickly.
Vision for the AISI as a statutory organisation
Expand beyond evaluations. The AISI’s next phase should have broader scope, including systemic safety, AI security, and governance for high-risk applications in areas like national security and defence.
Strengthen collaboration with the US. The San Francisco office of the UK AISI should be leveraged to maintain a strong presence in the US AI ecosystem. Partnerships with US intelligence and defence communities could open new avenues for collaboration in securing AI systems and addressing shared risks.
Secure infrastructure. Focus on securing data centres against cyberattacks and ensuring confidentiality in AI applications involving sensitive data. This can involve technical innovations like high-security clusters and confidential computing.
Attract top talent. Continue consolidating top AI talent within the AISI to strengthen expert advice on AI policy questions across government.
Avoid locking in the functions that may become irrelevant soon. Limit rigid structures by defining the AISI’s mandate with broad flexibility, allowing it to pivot and address emerging challenges in AI as it evolves rapidly.
Full interview
I. Industrial Strategy and areas of the UK’s competitive advantage in AI
Looking at the UK’s AI ecosystem, what areas of competitive advantage jump out at you?
The number one really has to be talent. London, or the Golden Triangle area more broadly, is probably the second-largest concentration of AI talent in the world, after San Francisco. It’s incredibly valuable to be able to draw upon this talent pool, particularly from leading universities, which are driving much of the innovation in AI startups in London.
Another competitive advantage, developed recently thanks to bodies like the AISI, is what I’d describe as state awareness or government capacity around AI within the Civil Service. This lets the government respond more effectively to rapid technological developments and capitalise on emerging opportunities. Ministers now receive good strategic advice that is technically well-informed, rather than a chorus of voices from academia. The AI Opportunities Action Plan is a good example.
Interestingly, another potential advantage lies in the shape of the UK’s economy. It’s somewhat tilted towards professional services and similar sectors, which are likely to experience significant productivity gains from technologies like AI agents [which can independently take actions to do complex tasks on behalf of users] over the next five years. The structure of the UK economy is more likely to benefit from AI rollouts and adoption compared to other countries’.
AI is expected to have a strong presence in the upcoming Industrial Strategy – the Green Paper mentions AI across several of the identified eight growth-driving sectors, not just in ’digital and technology’. In your view, how should AI be positioned within the upcoming Industrial Strategy? What deserves particular focus?
The main thing on my mind right now is the recent developments in language models, particularly around scaling and inference. With OpenAI’s recent releases, such as the o1 model, we’ve seen that increasing the compute used for generating text significantly boosts the model’s performance and capabilities in ways we hadn’t observed before. This illustrates the importance of having as much compute as possible to enable widespread rollout and adoption of AI systems across the economy.
The recent US export controls on both compute and model weights clearly increase the incentive for countries outside the US, including close US allies like the UK, to develop their own significant compute capacity. Over time, even in the US, there will likely be bottlenecks in the economic impact of rolling out AI due to high demand. For the UK to realise substantial productivity benefits from AI, it’s essential to invest in its own infrastructure.
That’s why I was optimistic when I saw the AI Opportunities Action Plan, which includes measures to increase energy availability for AI data centres and build more of them. I hope the Industrial Strategy will expand on these efforts.
In terms of AI adoption across key sectors, there are many levers the government can use to drive progress. Currently, many blockers to adoption are related to liability and risk concerns from enterprises – that is, how much risk, especially legal risk, they perceive in adopting AI technologies with high levels of automation. The government can play a role in addressing these concerns, particularly by offering clarity around the liability burden.
There is also a competitive advantage for the UK in sectors where it already leads strongly, such as biotech. This makes a compelling case for focusing more on AI applications in biotech, which is a highly promising area. For example, Anthropic’s CEO Dario Amodei recently highlighted the transformative potential of AI for bio-research in his essay on the future of AI. This is an area that might be slightly underappreciated by general AI commentators right now.
What areas can you see where the UK could be ahead of the game? What could be done policy-wise to set the right conditions for this to happen? What risks do we need to address to avoid missing these opportunities?
It all comes down to adoption as we see more and more capable AI systems automating various tasks. My mainline model for how capabilities will evolve over the next few years is that we’ll get to a point where we have something like a Google Chrome browser extension where you type in your task, and an AI agent completes it across many different websites, handling tasks of ten minutes in duration reliably, checking in with you for decisions or purchases. As models become more capable, this ten minute time horizon will keep expanding further and further.
This implies a need for significant inference-time compute to support highly capable models running constantly. The potential productivity benefits are enormous if we can achieve widespread adoption, particularly in professional services where these models could automate much of the grunt work. Any country that isn’t the US is about to experience huge concerns of being left behind economically – to stay competitive, they need to build more compute within their borders and ensure feasible access to it.
There is an underappreciated risk for so-called ‘third countries’ outside the US-China AI dynamic of losing access to the most capable AI systems. To stay ahead, they need to think about how to maintain access to these systems long term. This could involve building high-security data centres to reassure US companies they can deploy their best models, or to ensure the safe deployment of the best open-source models without risk of cyberattacks. It’s also crucial to have good state capacity to encourage adoption in appropriate ways.
We might see inequality in the adoption of AI systems due to limited access to compute in some countries or sectors, or varying appetites for risk. To reduce the risk of some countries or sectors lagging behind a lot in terms of benefits, you need to think carefully about how to ensure they can catch up and adopt quickly.
The UK is well-positioned for that. The AI Opportunities Action Plan demonstrates that the UK government is better informed about the future of AI than almost any other country outside of the US. Much of the plan is clearly written in anticipation of future AI capabilities, which is rare to see in other contexts.
You mentioned AI agents. Up to this point, consecutive Governments have taken a sector-based approach to AI regulation. Do agents change anything? Would AI governance need to account for agents in some particular way?
I don’t see any immediate need for adjustments. Right now, we have a general AI safety risk framework where sector-specific regulators deal with harms from downstream deployments, and the AISI, which isn’t a regulator but operates across all sectors, tests for general risks.
This framework should work fine for agents as well. The main difference is that we can expect to see potentially more relevant economic effects from agents across different sectors. That may motivate sector-specific regulators to put more effort into hiring AI talent internally.
II. Building the UK’s state capacity to deliver on national objectives in AI
The AISI is a remarkable example of building state capacity, and doing so quickly. And you were with it from the very beginning, starting with the Frontier AI Taskforce in DSIT.
What lessons have you learned from your experience at AISI that could be applied to getting other existing and future projects off the ground and scaling them effectively within DSIT, or government more broadly?
It’s a very interesting case study in how to do things well in government. Other people have written about this well, and I definitely agree with a lot of what Dominic Cummings says on this subject.
There are a few general principles I’ve noticed here. When you’re trying to build state capacity or launch a new initiative that needs to be impactful and move fast, you basically need to start a new team. If it’s an existing team, it’s probably too enmeshed in bureaucracy.
Secondly, you need significant political backing. In our case, we were fortunate to have great support from Henry de Zoete, who was then the Prime Minister’s Adviser on AI, and others in No. 10 and DSIT, particularly the minister at the time, Michelle Donelan. This means you can get around normal bureaucracy when needed, like hiring someone in a way that hasn’t been done before within the department.
You also need an incentive to perform well. This could be internal motivation from team members believing in the ideal or mission, a tight deadline like a Summit organised on very short notice, or competitive pressure from overlapping mandates with existing teams.
And finally, try to hire from outside government – people who are used to moving fast from industry and the startup world. That’s also effective.
There is an inevitable effect where a new, fast-moving, high-entropy team gradually gets pressed down by bureaucratic systems like HR, contracting, and finance. The general systemic incentive is to reduce fast-moving teams to a low-entropy state and make them more similar to the rest of government.
To counteract this, you need political backing and might need to start a new team or initiative if the first one becomes too slow. This explains why politicians often like to start new teams. There is an analogy here to the fractal startup model in industry. OpenAI scaled their ChatGPT team by creating conditions mimicking a pre-seed stage startup – totally new Google Drive, getting the new product team together in person five days a week, creating a separate office space – and that works surprisingly well.
In your view, is the AISI at a point when it’s time to create a new team within itself?
I wouldn’t go that far. They seem to be doing pretty well.
Where could the ‘fractal startup’ model be beneficial in the UK’s AI landscape?
If we were to launch Alan Turing Institute v2 with ten highly talented individuals and a budget of £10 million, challenging them to outperform the existing Turing Institute, would they? I suspect that with the right people, they would.
The Alan Turing Institute was originally conceived as a loose centre of an academic network, primarily coordinating research. While it did do some of this, it ended up spreading its bets too much, and there has not been that much impact from it. They have also struggled to keep pace with the rapid advancements in AI capabilities, especially in language models.
Given these challenges, there’s an argument for essentially restarting the Alan Turing Institute. If the old team has become quite bureaucratic or less successful in its mission, then start a new fast-moving initiative, give it some budget, hire a talented founding team, and see what happens.
III. The future of the AISI, AI safety market, and regulation
The previous Government focused on AI safety. The UK hosted the world’s first AI Safety Summit, and pioneered the AISI which has prompted the international network of AISIs to form. Ours remains the most well-resourced one. Do you think these efforts have given the UK a meaningful strategic advantage in AI?
Yes, I do, and it’s not just limited to safety. One of the key theories of change and motivations for the AISI from the beginning was not just to do pre-deployment or post-deployment testing between companies to improve model safety that way, but also to create a strong body of AI talent that is highly aware of the current and likely future capabilities of AI. This talent pool can be called upon at any time by ministers and others to advise on many aspects of AI policy.
I believe this has successfully happened. I’ve observed a consolidation of AI talent within DSIT into the AISI. This means there are many great people there who can advise very well on things like the implementation of the AI Opportunities Action Plan, or, as I did when I was there, on how the AI Research Resource should work, how to manage compute resources, or how to encourage adoption. These aren’t particularly safety-specific things, but the existence of the AISI gives the Government this large pool of talent and advice to draw upon. This is reassuring for ministers in many ways because they can ask questions and get answers from what is perceived as a more neutral source of expertise, compared to going to a think tank or external researchers.
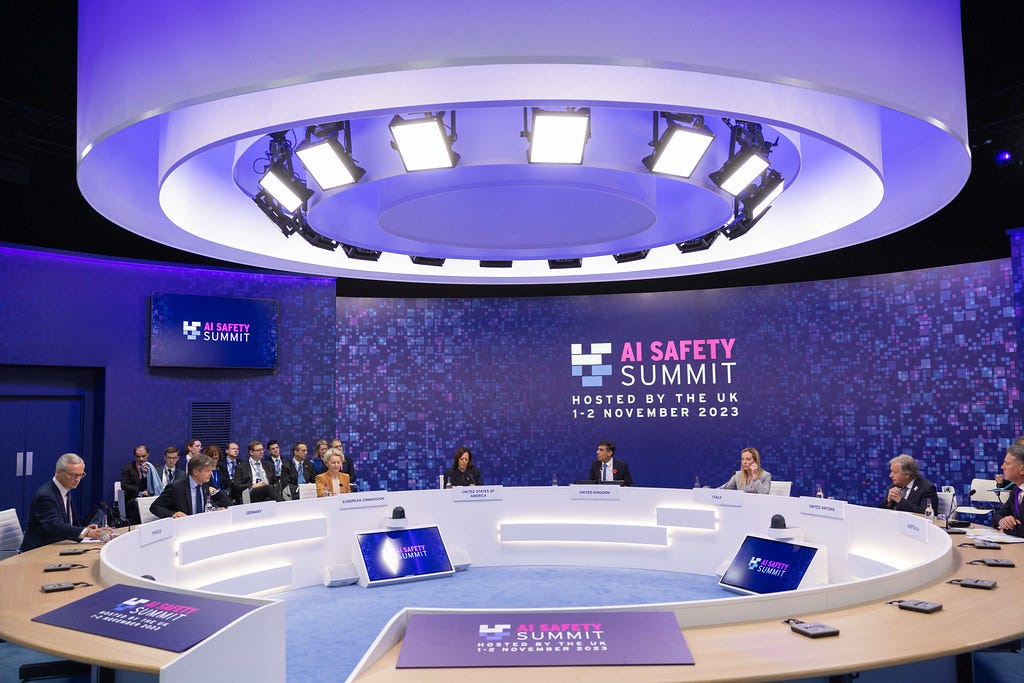
Would you say it is more of an advantage for the government, or for the national AI ecosystem as a whole?
It’s most easily seen as an advantage to the Government, but I’ve definitely also observed some trickle-down effects. For example, when people from the AISI advise on the implementation of the AI Research Resource and how its access scheme should work, it makes the AI Research Resource more effective because it’s able to draw upon this expertise more easily. This benefit then extends outward.
Essentially, the theory is that by drawing upon people who are very aware of the state of AI, you can build better policy, and it, in turn, becomes a strategic advantage for the country as a whole.
AI safety doesn’t end at the AISI. What could a broader AI safety market look like, and what does it need to thrive in the UK? How do you see the AISI’s role in this landscape? Doesn’t its evaluations [often called ‘evals’] capacity present a challenge to a third-party assessments ecosystem?
The AISI realistically is not going to have enough capacity to evaluate very context-specific downstream deployments of language models or agents, and nor should it. That’s not necessarily within its remit. Instead, it is to tackle these really large general risks, national security risks, autonomy risks, and others. I think there’s a place for both the AISI and an ecosystem of assurance for downstream deployments which can speed up adoption throughout the economy.
In terms of what role the AISI can play, it can hopefully just be a source of advice or develop tooling which is relevant to the development of downstream deployments. I was involved with the Inspect Evals project, which is an open-source evaluations library. That seems like exactly the kind of tool which might be useful to companies in this third-party assessment market.
What forms can an AI safety-focused startup take?
There are many pitches right now in this fairly crowded marketplace for startups focused on model evaluations and robustness.
One area for startups that I see as neglected right now is cybersecurity – building novel products based on cybersecurity expertise that can help, for example, secure AI agent deployments.
There are also many vulnerabilities in open-source software, and we’re increasingly reaching a stage where AI systems can be used to help secure these vulnerabilities because they’re becoming good enough at finding and fixing them.
I can see some startup potential in the direction of defensive use of AI. We see some of this with the Entrepreneur First (EF) defensive acceleration (def/acc) cohort.
Finally, there’s still another neglected area, I think, which is that if you’re a large company and you want to use an AI agent in some downstream deployment – let’s say in your hiring pipeline to automate some part of the process – then there are liability risks and other more prosaic risks that you might face. These risks might make you, as a large company, reluctant to face that legal risk. I think there’s a place for evaluation startups focused on assessing the specific context of downstream deployments. This is much less general than the work the AISI does, but it is potentially quite incentivised by the market, especially as we get more and more capable agents and more incentive to roll them out.
How are British startups doing in that space?
There are a bunch of existing startups in London just focused on language model evals-as-a-service. So that’s the area which is not neglected here. What I don’t see so much here yet, though EF might change it, is in the intersection of AI and cyber.
Do you think an AI safety market can really take off without mandatory safety testing?
I think in the long run, it’s unrealistic to expect a large ecosystem of third-party pre-deployment evaluations to emerge for the most capable models, especially those developed in the US. Those pre-deployment safety evaluations are most likely to be from governments or a very small number of existing evaluation research labs.
The key incentive is in downstream deployment, where I don’t think there needs to be any regulation. There’s already a strong market incentive driven by liability concerns. Companies will naturally want to assess risks, such as an AI agent in a hiring pipeline potentially producing outputs that cause legal risk for the company – these companies will need a mechanism to ensure they won’t get sued. This will likely emerge through AI insurance products and third-party assurance startups that can evaluate and certify AI systems, helping companies reduce their liability risk.
In terms of general safety testing, I see this consolidating over time to involve fewer external non-lab entities. The existing sector-based regulatory approach seems sufficient, essentially incentivising post-deployment safety.
Going back to what you mentioned about post-deployment safety testing, it sounds like the UK’s current sector-based approach to regulation makes sense.
Right, it’s essentially incentivising this post-deployment safety, so it works really nicely and makes sense. I don’t see much benefit in terms of meaningfully reducing risk through mandatory pre-deployment safety requirements or some form of frontier safety regulatory structure.
Similarly, I’m not optimistic that approaches like the EU AI Act will meaningfully reduce risk. There are many steps in the chain that need to occur for the mechanisms of the EU AI Act and its interaction with the Code of Practice in the AI Office to effectively motivate companies to proactively reduce risk. This is particularly challenging because many governments, including the UK and US, are already conducting pre-deployment safety testing. For general risks, there’s especially little incentive to motivate companies to comply, and there are ways to avoid the EU AI Act if a company is determined to do so.
In your view, is the AISI focusing on what it should be at the moment? Is there anything you would do differently in how it allocates resources, or what it prioritises? What opportunities for its impact remain untapped?
There are several potential AISI theories of change. Pre-deployment safety testing is just one approach, and I’ve always personally found it the least compelling. I’m much more attracted to the idea of building great state capacity in AI, and ensuring policymakers understand where the technology is at and where it’s going. Another good one is pursuing international collaborations, particularly with the US, on evaluations and technical AI research.
The Institute’s focus on evaluations made sense at the start, but it may have focused too much on this. AISI is aware of that, and I think that is why there is now some branching out into areas like systemic safety as they scale up.
It would be quite wise to focus more on AI security – specifically, securing training and deployment data centres against cyberattacks and working closely with the US through mechanisms like Five Eyes. AI security will become much more critical in the years to come, as I expect many capable cyber actors to want to attack highly capable AI systems.
Similarly, they should think more about the governance of AI applications in military and national security, which we should expect to see ramping up over the next few years, especially given emerging partnerships between AI companies and defence contractors like OpenAI and Anduril, or Anthropic and Palantir. It might be worthwhile for the AISI to get more involved in how the UK Government and military should think about AI applications in those areas. And there’s also room for more resources dedicated to understanding economic impact.
In a way, leading companies now seem to be converging on safety testing practices – they’re all building up evaluation teams and refining their work. Building up more and more evaluations is a less useful focus now than it was a year ago. The Institute has effectively accomplished its initial mission of developing a strong evaluation capability, so now it’s time to explore what’s next.
The initial laser focus on evaluations provided a clear organisational mission that allowed rapid progress. But now that product-market fit has been found, and it’s time to branch out.
The Government has committed to placing the AISI on a statutory footing. What opportunities and risks can you see with this, especially given how rapidly the sector is moving? How can the Government ensure that the Institute’s functions and role remain relevant in a year or two?
Placing the AISI on a statutory footing essentially means enshrining its existence in law, creating a permanent institution with long-term political stability. Historically, when a team is placed on a statutory footing, it typically means spinning out of its original department. For the AISI, this would mean becoming a more independent agency outside of DSIT.
There’s a potential risk of locking in the Institute’s form and responsibilities, especially given how rapidly AI technology evolves. The key is to define the Institute’s mandate with sufficiently broad language, focusing on high-level responsibilities around fundamental risks from the most capable AI systems. This would give UK leadership significant flexibility in how the Institute operates and adapts.
But this transition would come with some trade-offs. On the one hand, there will be less internal closeness to policymakers and DSIT staff, potentially introducing more friction in collaboration. On the other hand, becoming a more independent entity could offer advantages like greater freedom from DSIT’s bureaucratic hiring policies and contracting policies, which would let AISI move faster. The Institute would potentially gain more flexibility to develop its own capabilities and processes.
I understand that the UK AISI leverages the US AISI to be able to test the most advanced models from the US-based AI labs. At the same time, the future of the US AISI is uncertain, depending on what the new Trump Administration decides to do about it. Does this imply potential challenges for the UK AISI?
Yes, I think it definitely does. I shared some more detailed thoughts on this in a recent post about the potential implications of Trump’s election. In short, the US AISI’s implicit mandate as the central point for safety testing within the US government was partly dependent on political support from the Biden White House and the Department of Commerce. Much of the safety testing being done by the US government, not just within the US AISI, stems from Biden’s Executive Order. It’s important to note that the US AISI’s existence isn’t directly tied to the Executive Order, as it wasn’t created by it. So repealing the Executive Order won’t eliminate the Institute. I expect it to continue existing in some form, perhaps in a different department or with a slightly altered scope or focus. This means we can expect to see testing of frontier AI models for risks, robustness, and reliability become more decentralised or spread across various agencies within the US federal government.
This presents a challenge for the UK AISI because its agreement with the US is specifically through the US counterpart – it is a cooperative safety testing agreement between the two. If the US AISI is doing less testing overall, it potentially reduces the UK Institute’s involvement in the US ecosystem. This could also pose a challenge for the international network of AISIs that operates through the same channel.
With the San Francisco office of the UK AISI acting as a direct link to the US AI ecosystem, how do you see its role against that backdrop of uncertainty around the US approach?
Regardless of the US government dynamics, having a San Francisco office seems reasonable for the UK AISI, particularly from the perspective of bringing in more talent and expertise to the Institute.
One reason why I mentioned AI security and AI applications in military and national security earlier is that the UK-US national security partnership is quite robust. There are numerous channels between the intelligence communities that the UK AISI could potentially leverage to form more collaborations around the national security implications of AI.
IV. AI Research Resource compute strategy
As you mentioned earlier, you were involved in the AI Research Resource (AIRR). Why do you think AIRR was needed in the first place?
At the time, there was an obvious massive gap motivated by research computing needs. If you were a UK researcher at a university or academic lab around 2022, you’d be looking at increasingly capable language models and more intensive research requirements, but there were no university clusters even comparable to the smallest clusters of any company doing research in this area.
For context, I worked at Stability AI before joining the UK Government. We had a very large cluster of NVIDIA A100 GPUs, around 5,000 I believe, which was an order of magnitude larger than the biggest academic cluster at that time.
There was a clear need for more compute resources for research. The theory is that making much more computing power available for AI research would massively boost academic research, allowing primary investigators and professors to find ways to use it effectively. When researchers are greatly constrained by compute, their thinking becomes constrained, and they become less ambitious. They think of fewer out-of-the-box ideas with high potential. This is why industry labs ensure they provide abundant resources.
The AIRR was motivated by this need, and it’s a good pitch. But also, and I pushed for this when I was in government, it’s a great way to provide more resources to startups as well. I’m certainly very keen on allocating a decent portion of the resources to startups, and I hope that goes forward as the AIRR capacity expands.
The AI Opportunities Action Plan proposes a twenty-fold increase in the AIRR’s capacity. How sufficient do you think that is?
It depends on how it’s measured. Assuming the current AI Research Resource capacity is the baseline – where the Isambard cluster alone has 5,500 NVIDIA GH200 Grace-Hopper GPUs – a 20x increase would equate to about 110,000 GH200 equivalents, which could potentially be achieved with fewer physical chips using the next generation of NVIDIA GPUs. That would likely be enough to support almost all model training and academic research needs that the UK could feasibly want to do, plus a large portion of model training for startups.
When I was in government, I pushed for AI Research Resource access to be given to startups. If you’re going to increase compute capacity that much, then you really need to think about how to widen access to it for startups and other companies. Realistically, while it’s true that if you give academics compute they will think of ways to use it, I’m suspicious that there will actually be enough worthwhile purely academic research uses of it. With that much compute, large portions could also be reserved for important deployment use-cases rather than AI research, such as using the most capable AI agents to assist scientists in speeding up their work in key areas like biology or materials science, for example.
According to the AI Opportunities Action Plan, the AIRR will now operate under missions-focused programme directors who will oversee compute allocation. What are your thoughts about this strategic shift?
I quite like the mission-focused approach, fundamentally because it’s about making bets on particular directions, which I think is what the government should be doing with a large portion of this compute.
There’s a very common failure mode in large government compute projects. Some amount of money is allocated for compute, but then it either gets spread thinly over many clusters, or access to the large cluster gets spread thinly over many universities or labs. As a result, any one individual lab doesn’t get a significantly larger amount of compute than it had access to previously. This essentially wastes the entire investment. If you wanted to do an ambitious research project requiring training a model on a significant amount of compute, it’s unworkable if you split the compute ten ways across the UK. Therefore, I think making bets on some particular research direction or mission, with large fractions of compute allocated to a small number of initiatives, is the best way to allocate resources. As it says in the AI Opportunities Action Plan, spreading compute thinly doesn’t really work out at all.
What about the strategy of appointing programme directors? What do you think is needed for this approach to succeed?
I suspect that most large-scale AI research projects proposed by UK universities are probably not that useful. If you see some principal investigator proposing to use a million GPU hours on training a model – which is likely to end up as some domain-specific model that’s probably not going to be much better than existing open-source models – then this is effectively not a great allocation of compute.
I’m personally betting on the ability of DSIT, given the talent pool available within the AISI and elsewhere, to be well-informed about which research directions would be useful. In some cases, they might be better informed than the collective of UK academics or the Alan Turing Institute about the capabilities of cutting-edge systems and what research directions are useful.
I think the US National AI Research Resource [the UK AIRR’s equivalent stateside] has done well to involve some top, well-informed academics with good research taste.
To be honest, there are few of these people available in the UK university system. That’s why I was always a bit worried about the dynamics around AIRR. A potential failure mode is when someone who isn’t really a cutting-edge AI researcher at a university, or a professor with 30 years of experience but little experience in frontier AI development or research, is driving a lot of the research decisions. This could result in poor allocation of resources.
What else could the government do to realise the full potential of AIRR?
There is one particular compute project that could be quite useful to direct some of the AIRR to as it expands: a pilot high security AI cluster of decent size. The goal would be to have a testbed for solving many of the technical problems around building higher security AI clusters, both securing sensitive AI systems from highly-capable cyber actors, and also unlocking use-cases for applying AI to confidential or private data. This project could help build valuable technical expertise on handling these kinds of security challenges.
Another opportunity is skills development. Having this amount of compute available is an excellent way to train potential research engineers and provide hands-on experience with foundation model development. Currently, one of the main bottlenecks in the UK for skills growth in AI, which ultimately constrains the UK startup and research ecosystem, is the lack of sufficient compute for people to experiment with tasks like fine-tuning large language models at scale.
A focused talent programme could go a long way. Even a two-month boot camp, a serious GPU cluster and training time could potentially bring skilled Master’s graduates up to a level which would be great for doing proper frontier AI training.
What about an elephant in the room? A twenty-fold increase in compute capacity won’t appear in the UK overnight, especially given the constraints that need to be overcome to get supercomputers built. What are your thoughts on this?
Certainly, this much compute is going to be bottlenecked by energy. You’re going to have to think very carefully about the right sites and likely make some sacrifices on location to find the right energy viability. It’s probably not going to be possible to do it all with green energy either, because solar and wind are not baseload power sources, and their location constraints limit the options too much.
Also, as you say, it takes time to build data centres. It is possible to speed up construction to some degree – for example, Isambard-AI in Bristol, which is the first big cluster of NVIDIA GPUs for AI research in the AIRR, is a modular data centre. Essentially, different parts of the data centre are shipped in containers and then connected together. This approach makes it very fast to construct, but slightly less efficient in some ways. It’s not quite as ideal a setup as having a big warehouse with all GPUs inside.
V. Driving the AI Opportunities Action Plan with long-term foresight
If you were advising the DSIT Secretary of State Peter Kyle on AI policy right now, what would be your top advice?
Looking ahead to the next five years, the main high-level point is not to take for granted that US-aligned allies will get unfettered access to the most capable AI systems which could have the biggest benefits for the UK economy.
There will be many incentives, which also motivate many aspects of the AI Opportunities Action Plan, for compute sovereignty in various forms. The UK is in a good position and moving in the right direction. It’s important to keep in mind the substantial risk that widespread benefits from AI in terms of productivity could be severely bottlenecked by compute resources, which in turn could be constrained by energy.
Other potential bottlenecks to seeing widespread benefits include access to models and political engagement with the US on AI governance. On a strategic level, I would prefer the UK to align more closely with the US than with the EU here.
What do you think is the most interesting recommendation in the AI Opportunities Action Plan that we haven’t discussed yet?
It proposes to establish an internal headhunting capability on par with top AI firms to bring a small number of elite individuals to the UK. The aim is to recruit top talent for the AISI, the proposed UK Sovereign AI unit, other public AI labs, or UK-based companies.
This approach makes sense from an economic perspective. A significant amount of economic value is often created by a surprisingly small number of highly talented individuals who are the best researchers, engineers, founders, or leaders in their fields. Attracting even a relatively small number of these people to the UK could have substantial long-term economic benefits.
We ask all our guests the same closing question: what’s one interesting thing you’ve read or listened to recently that you’d like to share with our readers?
Try listening to Tyler Cowen’s recent podcast with Dwarkesh Patel, discussing the economic impacts of AI. It’s a nice contrast between Dwarkesh, who is very bullish, and Tyler, who is on the very sceptical end of the discussion.